Bill Rand Explains Big Data Analytics and Machine Learning in Today’s World
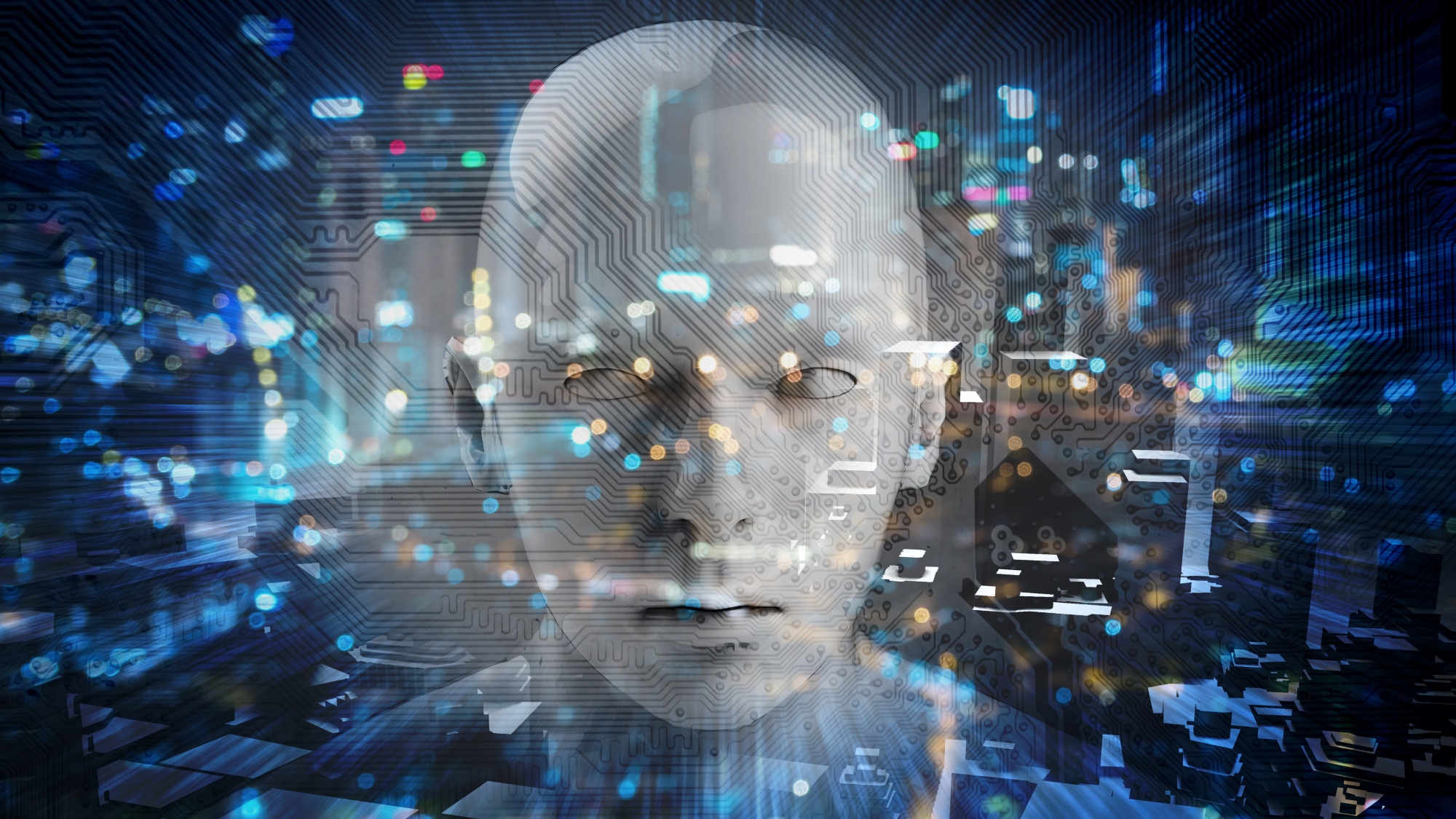
It’s no surprise that big data is changing the business world. Companies around the globe have access to more data than ever before but the question is… how can they use it efficiently? The answer is machine learning. And while it’s the next big step in technology for companies to utilize their big data, there’s still so much to be learned.
At Poole College, there’s no one who knows more about big data, machine learning and marketing analytics than Bill Rand. An associate professor of marketing, Rand has spent years examining the use of computational modeling techniques to help understand and analyze complex systems, such as the diffusion of information, organizational learning and economic markets.
On September 28, Rishika Rishika, director of the Master of Management, Marketing Analytics concentration (MMA) at Poole College, sat down with Rand for an eye-opening conversation about the current data landscape, trends and predictions for the future of the industry. Here are some of the highlights. (You can also watch the full conversation in the video below.)
*Note: Some of the responses have been edited for brevity and clarity.
We hear the term “big data” all the time. Can you tell us what it means?
Rand: Big data is an important point of transformation that’s happening in the business world. It often gets attention from the word “big” but I think that might be the least interesting part of it. There’s an old IBM definition that talks about big data through the lens of the three V’s – volume, velocity and variety – and I actually think that the variety aspect is the most interesting. Historically, business decision making was about using data they already have – that’s been collected in exactly the right format that they need – and then trying to figure out what to do with it. But variety means that we can now look at data that’s not stored in that way.
Today, we can look at text analytics or social media data and transform it into data that we can act on. For example, if we’re running a call center, we can look at text-to-speech translation and then analyze the data that comes out of it. That ability to transform unstructured data into structured data is one of the most important parts of the big data revolution that’s going on around us, and that’s really the crux of what will transform companies’ decision-making processes in the future.
You touched upon the notion of structured vs. unstructured data. Could you tell us what differentiates the two?
Rand: Structured data is basically anything that is already in some sort of organized format – typically, a relational database where you have rows and columns that describe the activities of a business or operation. Unstructured data is data that’s been accumulated, but you don’t have any way to directly draw insights from it. And so a lot of what we’ve been looking at, and what a lot of machine learning tools are doing, is being able to take unstructured data and transform it into a format that is more easily accessible to make business decisions. At Poole College, we do a lot of work with unstructured data – specifically within the Business Analytics and AI Initiative.
For the last decade, many major companies have invested in machine learning. But in recent years, it seems that everyone is talking about it. Can you tell us a bit more about what machine learning actually means?
Rand: Machine learning is the idea that you can take a data set from a company or organization, and you run a tool over it that attempts to learn patterns in the data. There’s a pipeline that I reference when discussing machine learning and analytics in general: DATA -> INFORMATION -> KNOWLEDGE -> WISDOM. We start with raw DATA that doesn’t have a particular application to a particular domain area. Then we get to INFORMATION, which is data that’s been collected into pools of information that are relevant to the topic we’re looking at. Beyond that, we start to think about KNOWLEDGE, and it’s the translation between information and knowledge (where we’ve identified patterns in the data that are useful) that is really where machine learning comes in. Finally, the most critical part is how to take that knowledge and transform it into WISDOM. So machine learning is really the transition from information to knowledge – how we take collected data, use it and understand the patterns that are present in it.
A classic example is shopping cart analysis, where stores use loyalty cards to track purchases and learn behavioral patterns for what customers will buy and when. That’s really what machine learning is all about – learning those models of behavior and patterns that are present in the data. There are a lot of tools to help with this, from simple linear regression to deep learning nets and random force that have become more advanced analysis tools.
Which market or industry will be most impacted by machine learning and big data?
Rand: I would say that those most likely to be impacted are those who have data that hasn’t traditionally been stored in structured formats. For instance, an apparel manufacturer. As they get better tools to be able to match a particular shape and description of dresses or shirts or ties, to particular desires that people have, it will better inform their operations and output. Another aspect that I’ve been working with is the law – specifically legal analytics tools that will help lawyers make better decisions about what kinds of arguments to pursue and what kinds of court cases to look at – and that’s an area that hasn’t been explored at all. Some of our friends at Campbell Law School are actually exploring those topics themselves.
I’m sure we’ve all seen the Tom Cruise movie “Minority Report.” Are we getting to that level of forecasting with big data?
Rand: So there are two aspects of that movie that are relevant to this discussion. One is the aspect of ever-present advertising and the other is predicting crimes before they happen. I don’t think we’re at either event yet, luckily! In all seriousness, we are likely to see more tailored advertising to our particular interests – but I don’t think it will become as pervasive as it is in the movie. In terms of the criminal predictions, there are just so many ethical and privacy issues to deal with – things we’re actually trying to address in the Business Analytics and AI Initiative. At what point are firms using too much data? Are there ways to use insights about your customers without going too far? That’s another area to think about as we approach better methods of predictive and prescriptive analytics.
An interesting fact is that Amazon actually patented a pre-purchase predictive tool that they say allows them to know what you’re going to buy before you buy it. It’s the reason they’re able to do one-day and one-hour shipping. The algorithms that make sure that the right goods are in the right places at the right times so they can get your purchases to you as soon as possible.
What types of software are used in machine learning?
Rand: Historically, most machine learning was done by computer scientists who were writing code from scratch. Today, more and more is being done in standardized data science formats – Python and R are the most dominant languages there. There’s also usually some sort of data science workspace that may wrap around that; Anaconda is a very popular one. For more advanced machine learning, JMP builds a more point-and-click type interface. And if you go all the way up, you have tools like Power BI and Tableau which are almost entirely click-driven interfaces that allow you to do machine learning on large-scale data sets. Over time, we’re going to continue to see providers make things more automated, turning machine analytics into a service where you pose a question and they find the answer… essentially the tools will just do it.
Machine learning and artificial intelligence (AI) are often used together. Is there a difference? Are they interchangeable?
Rand: For the vast majority of business applications there’s little difference between those terms, and personally, I think of machine learning as a subset of artificial intelligence. Machine learning is specifically about learning patterns in data that already exists, whereas AI is more general about how we allow computers to think and act like intelligent beings. So there are some parts of AI that aren’t related to machine learning – like giving a robotic interaction an emotional component. Machine learning is a specific idea which looks at the patterns that we’re seeing in the collected and how predictive it is. They often overlap in modern business domains.
How do you see the healthcare and business industries working on big data in the next 10 years?
Rand: The problem we’re facing in the healthcare industry right now is a deluge of data… we have more information than ever about our patients and disease states. What I would like to see in the next 10 years is the development of a system of tools that would allow these industries to bring all the data together to give doctors and operations managers the ability to access the information they need the moment that they need it.
An important topic that’s not really talked about is information needs. Do we have the right resources and information co-located in the right place so that the medical provider can provide the best solutions at that time? The ability to bring that information together and present it in a way that doesn’t overwhelm the healthcare provider is critically important. Many of the new AI techniques are really good at taking large amounts of data and figuring out what pieces of that data a doctor needs right now. Having tablets and tools that could adapt to healthcare providers’ uses would really revolutionize healthcare.
The other aspect that would be helpful is the advent of precision medicine, which is the idea that we take into account the variety of the human experience when deciding how to treat a particular patient.
As data analytics needs grow, many worry about the workload for professionals and if there are enough graduates to meet the demand. A recent article noted 90-hour work weeks for data analysts. What’s your perspective?
Rand: I’ll start by saying that we’re working to increase the number of graduates here at Poole College by increasing the programs we offer. Next, I’ll say that while a 90-hour work week sounds scary, it means that there’s going to be increased demand and salary increases for those who are interested in the space. Another important aspect to keep in mind is that as we increase the automation of data analytics, it will take less time to do the same amount of work. We should be automating as much as possible in data analytics – that’s really the part that doesn’t require much human intervention. As we better utilize automation tools, the marketing and risk analytics people will spend less time cleaning data and building machine learning models, allowing them more time to think about what questions they need to ask to make a better decision. I do think we’re headed in that direction. Will there always be jobs that require 90-hour work weeks? Yes, it’s inevitable. But I have many friends and colleagues working as data analysts right now and they are not working those types of hours… they have families and can balance a normal work life while still doing data analytics.
If you think about your own goals, where do you see yourself and your research going in the next 5-10 years? How do you see the field evolving?
Rand: Generally, all of my interests in the space go back to my undergrad days… my interests really haven’t changed. I was asking questions back then that are still big questions now. Fundamentally there are two areas that keep me up at night. One is diffusion of information, which I feel is focused around three questions – How do people find things out? What do they do with that information once they have it? How do they pass it around to others? In many ways, you could say that all of marketing is busy answering those questions. The other area is data-driven decision making. How can we provide tools to help people make better decisions, given the data they have about the decision they’re trying to make? These two areas are intimately linked. If we can study how people are making decisions now and how they’re looking at the information that’s coming in, we can start thinking about how they could make better decisions in that space and then give them the tools to do so.
Where do I want to go with this and how do I want to use machine learning to make this better? One aspect that’s growing and changing in this area is the ability to bring different types of data into context. Can we look at images the advertisers are presenting to customers and try to figure out what aspects of those images are making people click on a particular hotel room to book or dress they want to buy? Is there a way that we can change the layout and the aspects of those images to make them more informative for the consumer to allow them to make a better decision about purchasing a product? Beyond images, I’m really interested in video. We know that images and videos get 3-5 times the engagement on social media than text posts do, so what can we do to make better videos? It’s not a question that’s well understood. Are there keys or other patterns to video content production that are more useful for firms to use?
I think the answer to both of those is that we’ve got to use machine learning because there’s just too much raw data for a human to understand. We’ll still need someone to look at the analysis that those tools have done and figure out what they’re saying, but we’ll have to use machine learning to understand the rich environmental information systems that we’re dealing with nowadays.
Palantir, a data analytics company, recently bought thousands of gold bars. In data analytics they say that your predictions should always be wrong but should be close enough. Should we be afraid about a market crash or black swan event?
Rand: Palantir historically has steered away from traditional machine learning approaches and has looked more at rule-based approaches, so I don’t know if looking at what they are doing is necessarily the best reflection of what we’re talking about today. I’m not afraid of a large market crash or a black swan event. These things happen and we should try and keep in mind that that’s really a question of risk. We’re talking a lot about marketing analytics today, but another project we’re starting up soon at NC State is a risk and analytics degree that will allow you to use data to better assess risk – things like black swan events. I’m personally a big fan of data and I think you should always trust it, but you should be wary about where it’s coming from and who’s collected it.
- Categories: